In today’s data-driven world, the proliferation of digital information has given rise to the era of big data. Big data refers to massive volumes of structured and unstructured data that inundate businesses on a daily basis. To harness the potential of this data deluge, organizations are increasingly turning to big data analytics—a process of examining large and varied data sets to uncover hidden patterns, correlations, and other insights.
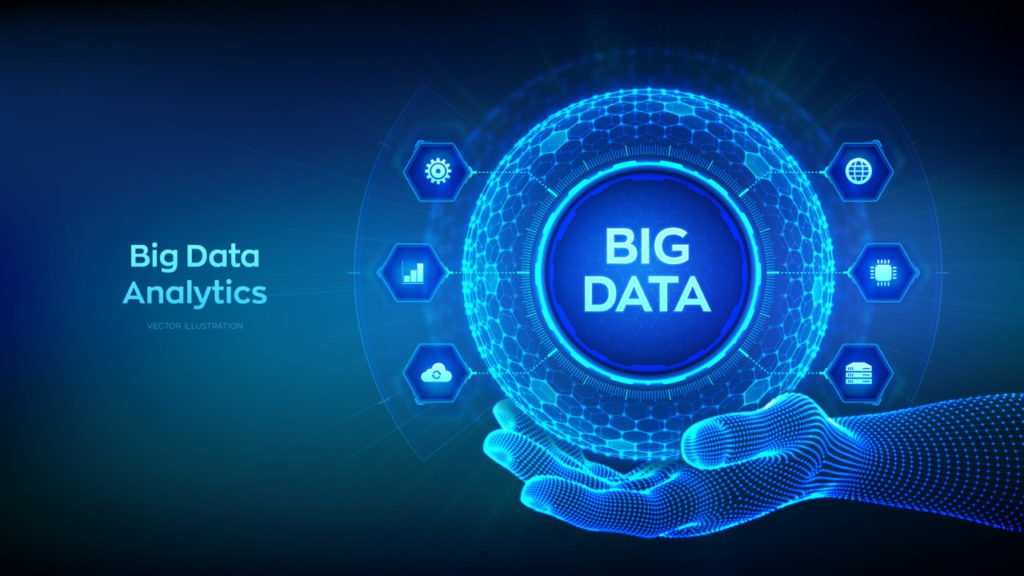
Understanding Big Data Analytics
Big data analytics encompasses a range of techniques and technologies designed to handle the complexities of massive data sets. Traditional data processing applications are often inadequate for managing big data due to its volume, velocity, and variety. Big data analytics tools and platforms are therefore equipped with capabilities to process, store, and analyze data efficiently.
Key Components of Big Data Analytics
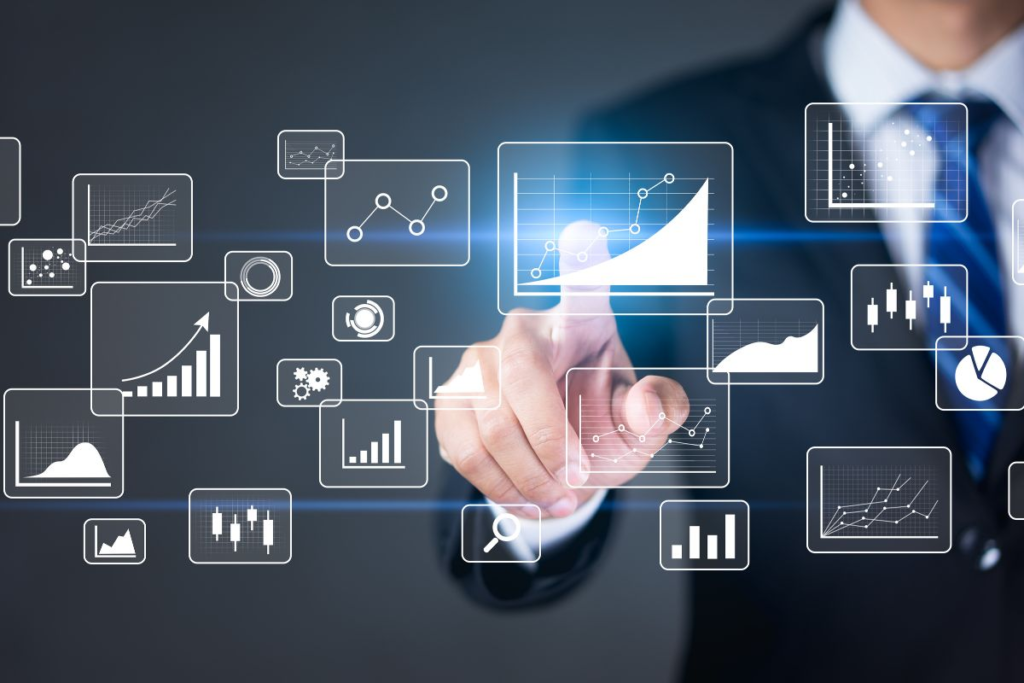
- Data Collection and Integration: The first step in big data analytics involves aggregating data from various sources, which may include social media platforms, IoT (Internet of Things) devices, transaction records, and more. Data integration ensures that disparate data sets are unified into a single coherent format suitable for analysis.
- Data Storage and Management: Big data storage solutions such as data lakes and distributed file systems (e.g., Hadoop Distributed File System) are designed to store vast amounts of data across multiple servers. These platforms provide scalability and fault tolerance, enabling organizations to manage petabytes of data effectively.
- Data Processing and Analysis: Big data analytics employs advanced processing techniques such as parallel computing and distributed processing frameworks (e.g., Apache Spark) to handle computations across large data sets. This enables rapid querying, real-time analytics, and complex data transformations.
- Data Visualization and Interpretation: Once data is processed, visualization tools and techniques are used to present findings in a meaningful way. Data visualization not only facilitates understanding but also helps stakeholders identify trends, outliers, and actionable insights from complex data sets.
Applications of Big Data Analytics
Big data analytics has transformative potential across various industries:
- Retail and E-commerce: Analyzing customer behavior and purchase patterns to personalize marketing campaigns and optimize inventory management.
- Healthcare: Leveraging patient data to improve diagnosis accuracy, predict epidemics, and enhance treatment outcomes through personalized medicine.
- Finance: Detecting fraudulent transactions, optimizing investment strategies, and assessing credit risks based on extensive data analysis.
- Manufacturing: Enhancing operational efficiency by monitoring equipment performance, predicting maintenance needs, and optimizing supply chain logistics.
Challenges and Considerations
Despite its potential benefits, implementing big data analytics poses several challenges:
- Data Privacy and Security: Safeguarding sensitive information and ensuring compliance with data protection regulations (e.g., GDPR, CCPA).
- Skill Shortage: A shortage of data scientists and analysts with expertise in big data technologies and methodologies.
- Infrastructure Costs: Investing in scalable infrastructure and cloud services to support big data storage and processing needs.
Future Trends
The future of big data analytics is poised for continued evolution with advancements in AI and machine learning algorithms. These technologies will further automate data analysis processes, enabling more accurate predictive modeling and real-time decision-making.
Conclusion
In conclusion, big data analytics represents a paradigm shift in how organizations derive value from vast data sets. By leveraging advanced technologies and analytical techniques, businesses can gain actionable insights, improve operational efficiency, and drive innovation across various sectors. As big data continues to grow in volume and complexity, the ability to effectively harness and analyze this wealth of information will be a crucial determinant of success in the digital age.